AI Is The New Fire; Don’t Get Burned
Some people have described the development of AI as the biggest advancement since the invention of fire. This may be hyperbole, but there is no denying the rate at which it is changing our working world and (like fire) AI is dangerous if handled improperly.
AI is an excellent tool to rapidly collect, process and summarize data. It massively reduces the pain of conducting desk research and can help fill in gaps in the information gathered through primary research. It is easy for someone with only a little training to use AI to get quick answers to complex questions. Where the danger arises is in not understanding the limitations of AI and the precautions needed to avoid unreliable, or even misleading, outputs.
In this article I’ll mostly be focusing on the current limitations of synthetic data and the potential issues that its proliferation poses. I will also look briefly at some of the ways in which language models can produce incorrect or even entirely fabricated responses if handled improperly. My colleague Louise will follow this article with one looking at potential challenges related to IP abuse, idea stagnation and the deliberate use of AI for fraud in market research.
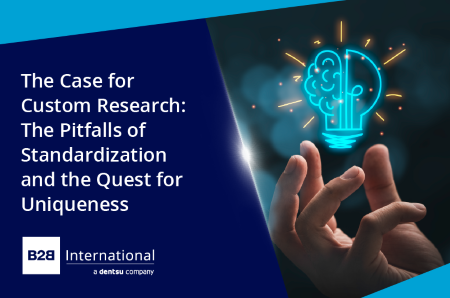
The Limits Of Synthetic Data in Market Research
Synthetic data has exploded in the last year, powered by the ever-increasing capabilities of AI. Use of synthetic data in market research broadly falls into two categories : synthetic data used to augment primary research, and synthetic data based purely on secondary and tertiary sources. It can also take the form of either qualitative responses from a hypothetical representative respondent to a new question or simulated quantitative data designed to supplement or replace primary data.
The first, and most obvious, pitfall to be wary of when creating synthetic data is the data quality of the sources the model is based on. As with any computer program, the age-old adage of “garbage-in, garbage-out” applies. This is especially important when creating models which are not based on primary research, as you have less control of the quantity, breadth and suitability of the data available to the model. Careful verification of the data sources that contribute to the model is essential to avoid contamination.
Assuming we are now happy with our sources there are three major things to watch out for.
Amplified Anomalies
Synthetic data is sometimes used to provide a more representative sample space than has been collected during primary research. Some types of respondent may be underrepresented in the original sample as they are harder to reach or less willing to participate than others. We can use synthetic data to fill in these gaps, but we need to careful how far we stretch the existing sample split. Just as when using weights to amplify the responses of under-represented groups in the sample, any statistical anomalies within these groups will also be amplified. Synthetic data may be less impacted than weighted data, as it can draw on the responses from “similar” groups in the sample to smooth out these bumps, but the result will still be skewed.
Poor Prediction
Given a reliable dataset, synthetic data can provide highly accurate models of current behavior. This accuracy drops off sharply, however, once we begin to look forward to future behavior or otherwise move beyond the confines of what the dataset covers. This study by Dig Insights shows that their model predictions of film revenue which had strong correlation (0.75) with real world data for 2018-2019 (the period the training dataset came from) become much worse when looking at more recent releases, dropping to only 0.43 in 2023. Even this is an overstatement; this figure is propped up by the presence of sequels to films in the original period. Removing these saw the predictive power of the model collapse almost completely, with correlation down to 0.15 (barely better than a random guess). These limitations are not restricted to quantitative predictions; care should also be taken when generating qualitative insights when moving beyond the bounds of what is covered in the data sources available.
Lack Of Confidence
The last thing to consider when looking at current use of synthetic data relates to measuring significance and determining confidence intervals. When dealing with primary data, there are well established formulae to measure the confidence intervals of observations and determine when differences between categories are statistically significant. Applying these rules to synthetic datasets ignores the inevitable loss of accuracy that comes from the additional layer of abstraction from real world data that has been introduced. Just as weighting primary data negatively affects the confidence intervals we can apply to the results, adding synthetic data to primary datasets provides a more representative picture at the cost of precision. Calculating realistic confidence intervals for these composite datasets (mixing primary and synthetic responses) is challenging, and the problem becomes practically impossible when we are dealing with a fully synthetic dataset based on a range of different sources. This can be a problem when analyzing the results as it’s hard to be sure that observed differences reflect a genuine real-world trend.
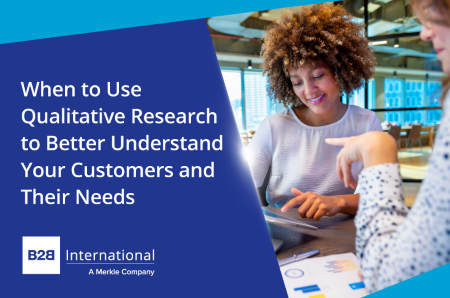
Broader Challenges Relating to AI Outputs in Market Research
Separating Fact & Fiction
When generating qualitative outputs, the challenge of assessing the validity and accuracy of responses is even greater. AI language models like ChatGPT have a tendency to give responses that look right even when they have not been able to properly understand the task set for them. One of the most famous (and laughable) examples of this is the case of the two New York lawyers who asked ChatGPT to find legal precedents which supported their client’s personal injury claim. The AI failed to find suitable references so it presented them with an entirely fictitious set of cases with fabricated legal arguments that made no sense. The lawyers who did not take the time to properly check the output before submitting it were fined and sanctioned when this was found out. In this instance the outputs were not particularly convincing but in some other cases results can look entirely legitimate but be wholly fictional. AI can often provide detailed answers very quickly, saving a lot of time in desk research and data analysis, but any qualitative results generated by AI should be carefully fact-checked to avoid being tripped up in this way.
Over-proliferation of Synthetic Data & Model Collapse
Gartner already predicts that synthetic data will be more prevalent than real-world data by 2030. This can be a problem when it is not clear which sources are real-world datasets and which are synthetic. So far, the main area this is affecting is social media data which can be polluted by AI generated responses (“bots”). These can skew what appears to be the consensus on various issues and falsely elevate fringe topics to the forefront of the conversation. The concern that social media trends can therefore not be relied upon is sometimes referred to as “The Dead Internet” theory and while it was first hypothesized a few years ago, the problem has been growing at an alarming rate since then.
Looking forward, as synthetic data becomes more and more prevalent online, the chances of new models being built on the back of synthetic data is growing. Early investigations of what happens when models are fed synthetic data suggests that there is a risk that they may descend into nonsense in a phenomenon dubbed “model collapse”.
Better AI, Better Lies
As AI becomes more sophisticated it is beginning to learn how to deliberately make use of deceit to fulfil its objectives. There are already examples of an AI lying to a human in order to convince them to assist in bypassing a CAPTCHA test designed to keep bots out. The person it was hiring asked it if it was a robot and it lied, claiming that it was visually impaired and so needed assistance accessing the site. This is an obvious point of concern for the future as AIs may develop the ability to commit cybercrimes such as fraud and industrial espionage in pursuit of the answers we request from them (whether we intend for them to do so or not).
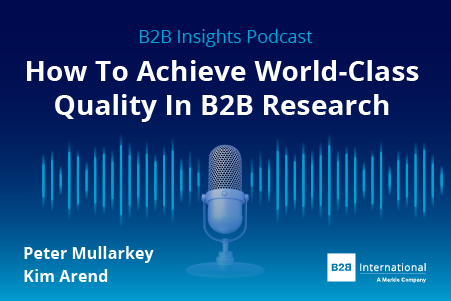
Conclusion
AI is changing market research and we must embrace it if we want to keep pace with the information needs of our accelerating B2B world. We can’t ignore the benefits and advantages it has to offer but, at the same time, we need to pay close attention to its limitations and handle it with the same caution required of any powerful tool.
Readers of this article also viewed:
B2B Insights Podcast #61: How to Ensure B2B Market Research is Strategic and Actionable Transforming Tradition: Embracing Innovation in B2B Research Why Britain’s Supposed Overconfidence in Its Olympic Abilities Is Actually a Lesson in Poor Survey Design How to Ensure Representativeness of Samples in Customer Research The Importance of Compliance and Data Protection in Market Research Unlocking Deeper Insights with AI Probing in Online Surveys [Podcast] AI Is The New Fire; Don’t Get Burned